Data Maturation Represents the Essential Reason for Deploying Machine Learning Today
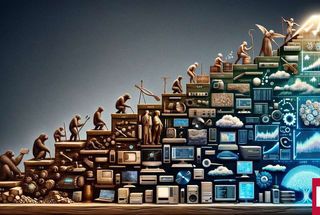
From the recent articles that Oracle Hospitality has published on both how machine learning (ML) works as well as some of its key applications, there is a single term that all hoteliers should remember when deciding how to commercialize this type of artificial intelligence (AI). This term is ‘data maturation’ and, as the name suggests, you need time to let the ML occur. You need to give the computers time to swift through the reams of training data to test variables and find the patterns that will then lead to the algorithms and models to advance business goals.
As with so many other aspects of hotel operations (and life for that matter!), time is the limiting resource. Significantly, we must remain cognizant of the large gap in learning between machines and humans because this difference can often twist our judgment about how quickly AI applications can be brought to fruition for a property or companywide.
From what we understand about consciousness, the knowing ape that is homo sapiens learns largely by causal inference. As a crude example of this contrast, let’s say you encounter an ash-ridden husk of a burnt-down house. You will very likely know intuitively that a fire was the culprit after only one occasion of this and without directly witnessing the fire itself.
Computers learn about correlation not causation. Without enough datapoints on this current ash-ridden house and other similar instances from the past, the machine cannot say with a high degree of confidence if it was an unseen fire that burned the house down or if the house first collapsed via another mechanism and the ash formed thereafter.
What computers need to get from the 0% to 85%, then to 95% and 99.999% (the ‘five nines’ as they call it) confidence intervals are observations. It needs to analyze different variables across as many instances as possible in order to develop a probabilistic model of how the world works, and then it needs to test and retest that model in order to refine how well it fits with real world outcomes.
Just as a child doesn’t become a mature adult overnight, from this previous sentence two important actions are implied:
- Deeper data connections: The more fields of datapoints the AI has that are associated with a given observation, the better it can evaluate hidden patterns amongst the vastness of numbers in order to build more accurate algorithms and models. Besides other key benefits of integrating various systems, the use cases for ML mean that APIs, CDPs and other unified platforms should remain a top priority for a hotel’s commercial strategy.
- Multivariate testing: Better data interfaces will yield more characteristics around a given observation, but the machine also needs more observations overall. Apart from the size of the initial training data, to learn and become increasingly accurate, the machine needs to A/B test and examine how people respond to its current modeling. Because this requires guests or customers to interact with measurable aspects of a hotel such as the website or mobile app, the more time that passes, the more physical interactions the machine can use to hone the model.
It’s this last part that drives the point home for why you need to develop a plan for deploying ML now and thinking in terms of data maturation over the long run.
To close with an example from Nor1 and how data maturation affects pre-arrival upselling revenues, lets say your hotel has designed its pre-stay funnel to include an ‘upgrade your stay’ confirmation email that’s sent out upon reservation and also seven days out from arrival. While the days out part is something upon itself that can be tested by the computer in order to optimize open rate and conversions, for simplicity we’re going to start from upon landing within the upselling platform.
Let’s say you present the user with three basic offers: add breakfast (F&B), upgrade to a suite (rooms) or purchase a spa voucher (wellness). At the start of ML deployment, all three offers are presented equally in the first, second and third positions, with customer #1 seeing F&B in the top left, rooms in the middle and wellness in the top right, while customer #2 might see rooms in the top left, wellness in the middle and F&B in the top right.
If customer #1 chooses to add breakfast, is this because they wanted breakfast or because the F&B offer was in the first position? If customer #2 also chooses to add breakfast when it appears in the third position, is this enough evidence to determine that the F&B offer is what guests want or is the sample size too small?
At this point, there are so many variables, obvious or otherwise, to make a causal determination. What if both guests just want some form of food offer while onsite and don’t care if it’s breakfast, lunch, dinner or a ubiquitous food voucher, but they chose breakfast because it was the only F&B option? What if the photography for the breakfast offer was palpably more colorful and drew the eyes toward it better than the other two? What if the breakfast offer was significantly cheaper than the other two and it was chosen because of its inexpensiveness? What if both customers entered the upselling platform right before dinnertime around 5pm and it was their hunger that was driving their decision?
Even with only three offers, there are too many variables to tease out any correlation, let alone causation, at this point. The only way to make any sense around these questions is to test, test and retest. And because all your potential guests aren’t going to visit your website or open a prearrival email all of sudden once you have your ML tool in place, it will take time for the observations to accumulate and for the model to mature, so best start accumulating now!